Opinion
Big Data and the Rise of Machines in Financial Markets
—
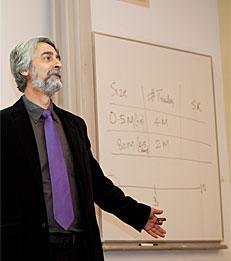

What if the entire data trail of the stock market were available to everyone after the end of a trading period, such as a day or a week?
By Vasant Dhar
During the most recent fanning of the flames against high-frequency trading (HFT), driven by the new book Flash Boys, Michael Lewis writes that a certain regulation (called Reg NMS) favors “a small class of insiders with the resources to create speed.” Indeed, much has been made of the speed advantages of high-frequency traders and whether these are “fair.” Some observers have even gone so far as to suggest more regulation to eliminate HFT and its supposed advantages! This kind of thinking is premature, especially without looking at the data. In an op–ed I wrote for CNBC during the latest furor over HFT, I clarified that speed is necessary to generate advantages in this business, but that the real advantage has been accrued through investment in big data and analytics.1
I exploited the slow stock market for several years through clever algorithms that used high-frequency data to identify short-term patterns in prices. The program had on average one losing day a month. Ironically, Reg NMS unceremoniously eliminated my advantage and conferred it on others who studied the regulation carefully, particularly the incentives it created for the different market participants and their behaviors manifested in the data.
The key to exploiting big data is to ask the right questions. In my recent article on data science and prediction,2 I illustrated this with a healthcare example, but finance is no different. The torrent of data emanating from financial markets—such as quotes, orders, trades, cancellations, prices, and messages—is enormous. Adding to this complexity is the fact that securities trade on multiple exchanges simultaneously, with each marketplace offering different incentives for orders being routed to them and regulation such as Reg NMS mandating that an order must be routed to the marketplace where it will receive the best prevailing price* (lowest for buys and highest for sells). When combined with news releases and economic data, the fire hose of information is overwhelming to all but the most analytically astute. A player with the ability to discover buried, but exploitable, patterns is at a huge advantage over the significant majority who are not well positioned to find them.
Read the full article as published in Big Data.
___
Vasant Dhar is Professor and Head of the Information Systems Group and Co-Director of Stern's Center for Business Analytics.
Follow @VasantDhar on Twitter
I exploited the slow stock market for several years through clever algorithms that used high-frequency data to identify short-term patterns in prices. The program had on average one losing day a month. Ironically, Reg NMS unceremoniously eliminated my advantage and conferred it on others who studied the regulation carefully, particularly the incentives it created for the different market participants and their behaviors manifested in the data.
The key to exploiting big data is to ask the right questions. In my recent article on data science and prediction,2 I illustrated this with a healthcare example, but finance is no different. The torrent of data emanating from financial markets—such as quotes, orders, trades, cancellations, prices, and messages—is enormous. Adding to this complexity is the fact that securities trade on multiple exchanges simultaneously, with each marketplace offering different incentives for orders being routed to them and regulation such as Reg NMS mandating that an order must be routed to the marketplace where it will receive the best prevailing price* (lowest for buys and highest for sells). When combined with news releases and economic data, the fire hose of information is overwhelming to all but the most analytically astute. A player with the ability to discover buried, but exploitable, patterns is at a huge advantage over the significant majority who are not well positioned to find them.
Read the full article as published in Big Data.
___
Vasant Dhar is Professor and Head of the Information Systems Group and Co-Director of Stern's Center for Business Analytics.
Follow @VasantDhar on Twitter