AI Tool Successfully Responds to Patient Questions in Electronic Health Record
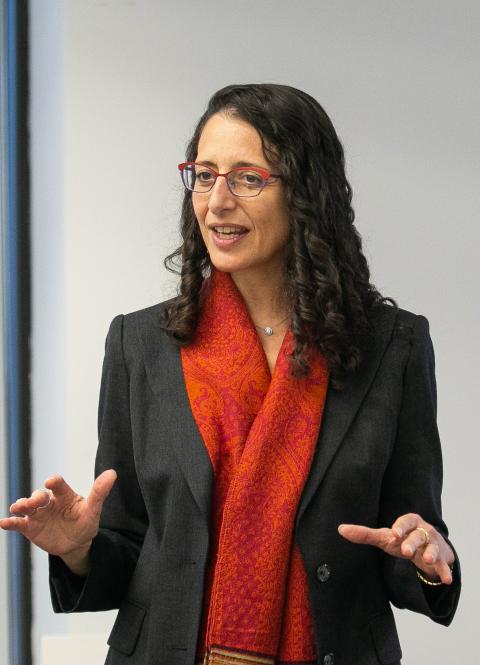
As part of a nationwide trend that occurred during the pandemic, many more of NYU Langone Health’s patients started using electronic health record (EHR) tools to ask their doctors questions, refill prescriptions, and review test results.
A new study entitled, “Large Language Model–Based Responses to Patients’ In-Basket Messages,” co-authored by NYU Stern Professor Batia Mishan Wiesenfeld, NYU Grossman School of Medicine Professor William Small, as well as other researchers at NYU Grossman and NYU Tandon, shows that an AI tool can draft responses to patients’ EHR queries as accurately as their human healthcare professionals, and with greater perceived “empathy.” The findings highlight these tools’ potential to dramatically reduce physicians’ burden while improving their communication with patients, as long as human providers review AI drafts before they are sent.
Additional study authors from NYU Langone were Devin Mann, Beatrix Brandfield-Harvey; Zoe Jonassen; Soumik Mandal; Elizabeth R. Stevens; Vincent J. Major; Erin Lostraglio; Adam C. Szerencsy; Simon A. Jones; Yindalon Aphinyanaphongs; and Stephen B. Johnson. Oded Nov in the NYU Tandon School of Engineering is also credited as a co-author.
Although physicians have always dedicated time to managing EHR messages, they saw a more than 30 percent annual increase in recent years in the number of messages received daily, according to an article by Paul A. Testa, chief medical information officer at NYU Langone. Dr. Testa wrote that it is not uncommon for physicians to receive more than 150 messages per day. With health systems not designed to handle this kind of traffic, physicians ended up filling the gap, spending long hours after work sifting through messages. This burden is cited as a reason that half of physicians report burnout.
NYU Langone has been testing the capabilities of generative artificial intelligence (genAI), in which computer algorithms develop likely options for the next word in any sentence based on how people have used words in context on the internet. A result of this next-word prediction is that genAI chatbots can reply to questions in convincing, human-like language. NYU Langone in 2023 licensed “a private instance” of GPT-4, the latest relative of the famous chatGPT chatbot, which let physicians experiment using real patient data while still adhering to data privacy rules.
Published online July 16 in JAMA Network Open, the research examined draft responses generated by GPT-4 to patients’ queries, asking primary care physicians to compare them to the actual human responses to those messages.
“Our results suggest that chatbots could reduce the workload of care providers by enabling efficient and empathetic responses to patients’ concerns,” said lead study author William Small, a clinical assistant professor in the Department of Medicine at NYU Grossman School of Medicine. “We found that EHR-integrated AI chatbots that use patient-specific data can draft messages similar in quality to human providers.”
For the study, 16 primary care physicians rated 344 randomly assigned pairs of AI and human responses to patient messages on accuracy, relevance, completeness, and tone, and indicated if they would use the AI response as a first draft, or have to start from scratch in writing the patient message. It was a blinded study, so physicians did not know whether the responses they were reviewing were generated by humans or the AI tool.
The research team found that the accuracy, completeness, and relevance of generative AI and human providers responses did not differ statistically. Generative AI responses outperformed human providers in terms of understandability and tone by 9.5 percent. Further, the AI responses were more than twice as likely (125 percent more likely) to be considered empathetic and 62 percent more likely to use language that conveyed positivity (potentially related to hopefulness) and affiliation (“we are in this together”).
On the other hand, AI responses were also 38 percent longer and 31 percent more likely to use complex language, so further training of the tool is needed, the researchers say. While humans responded to patient queries at a sixth-grade level, AI was writing at an eighth-grade level, according to a standard measure of readability called the Flesch Kincaid score.
“GenAI drafts are a double-edged sword: they are surprisingly competent and, contrary to the stereotype of impersonal machines, they are good at conveying sensitivity. But they are not a panacea,” explains Wiesenfeld. “At least in the short term, if they are longer and more complex to read they could actually add to workload,” she warns.
The study was funded by grants from the National Science Foundation.
___
This article was adapted from a piece written by NYU Langone Health. See the original publication here.